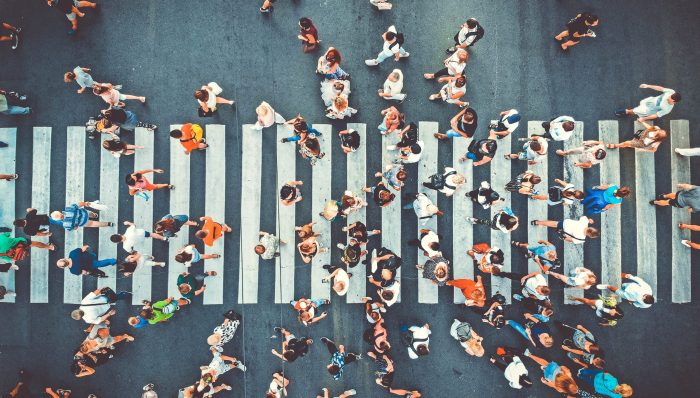
June 2023
Starting Small with Big Data
Early in the COVID-19 pandemic, the Public Health Agency of Canada (PHAC/the Agency) made use of innovative data sources and private sector expertise to inform the response to a fast-growing public health crisis. The Agency contracted with several big data providers and, with other government departments, began the work of making sense of the data.
At the outset, there was no dedicated team of experts at the Agency to explore this type of data for public health use. Instead, early work involved a small team with a keen interest and support from their leadership to engage with epidemiologists from across PHAC. The diverse expertise gained through collaboration with COVID surveillance leads and their partners drove much of the file’s early direction.
Building on this work, the Discovery Team was created to help lead in developing capacity for use of non-traditional data within the Agency. The team acquired licensed access to aggregated, de-identified mobile device data. This marked the beginning of a deeper dive into the potential value and uses of big data to support the pandemic response.
New work with novel data in the high-pressure context of a pandemic was challenging for the team. Naturally, there was much to learn about the data and how they could be put to use by public health in the context of the COVID-19 pandemic. But this work would also spark innovation, growth and the development of new skills within the team.
This case study is one team’s story and not intended as an endorsement of big data, nor a guide to using mobile device data. However, the team’s experience may help others understand options, opportunities and some promising ways forward for public health in this fast-growing area.
About Mobility Data
The widespread use of mobile phones has created large quantities of human behavioural data recognized as a rich source of information on population movement, with a variety of applications in different fields, including public health (1). Understanding the potential of these data for public health begins with understanding some data types and their features.
Two common types of mobility data that can be derived from mobile devices are operator data and crowdsourced data. Operator data are collected by cellular services providers based on regular device connections to nearby cell towers or when calls, messages or emails are transmitted. Crowdsourced data provide geographic information determined by the location of a mobile device receiving global positioning system (GPS) signals and collected from participating applications on users’ devices when they have turned location service on. The two types of data differ for certain features (see table on page 3) and both have strengths and limitations, depending on how they will be used.
The Discovery Team
The Discovery Team is a unit within the Public Health Agency of Canada that supports data analysis and builds capacity for use of novel data.
The team applies a public health perspective and brings both policy and technical expertise to the provision of several specialized services, including support for data acquisition, assessment of novel data sources, and the design and development of systems that collect, store and integrate data in visualization and analytical tools.
The team’s application of non-traditional data sources to support public health priorities is informed by research, consultation and privacy requirements. They lead the development of needs assessments, business cases, and stakeholder and user engagements.
Through recommendations to senior management, the Discovery Team supports the use of novel data and methodologies across the Agency.
The Discovery Team considered these two types of data for the kinds of analyses they could support. Both types of data allow for similar types of analysis at a population level, including general movement trends, connectivity analysis, which describes the amount of movement between communities, and points of interest analysis, which indicates patterns in people’s activities related to certain locations, such as visits to grocery stores or hospitals.
Although their analytical potential is similar, the geospatial specificity of location information provided by crowdsourced and operator data differ, placing some limitations on analysis. Crowdsourced data use geographic coordinates to determine the location of devices in a sample. Through complex aggregation methods, the de-identified but specific location data points in crowdsourced data can show changes in regional movement trends and patterns. Operator data rely on cell tower density, which differs across geographies (i.e. urban centres have more densely spaced towers compared to rural and remote areas). For sparsely populated areas with few cell towers, operator data are limited in how accurately they can reflect the locations of devices in a data sample. This can place some limitations on analysis of population movements relative to points of interest for those areas.
The Discovery Team also viewed their data through a public health ethics lens. Ethical values and principles of public health govern how the Agency delivers on its mandate to promote and protect the health of Canadians, including consideration of data privacy and security. From the start, the team critically appraised the data for ethical considerations and received advice from internal legal and privacy experts. As well, PHAC’s contracted data providers had their own practices and adhered to policies that protect the personal information of Canadians. The spotlight piece from BlueDot Inc. (see page 4) provides one contractor’s perspective on data privacy and security considerations.1
1. The inclusion of content from BlueDot Inc. does not represent an endorsement or recommendation by the Public Health Agency of Canada.
Mobility Data Types and Features
Data Type | Geospatial Accuracy | Population Representation | Data Collection | Data Access Time | Types of Analysis |
---|---|---|---|---|---|
Crowdsourced Data (app-based) | Latitude, longitude positioning with near-precise accuracy across all provinces and territories | Sample size dependent on the number of app users Sample size fluctuates daily or weekly | Triggered when users have opted in to share their location on apps (i.e. restaurant, shopping apps) Location captured when app is in use and enabled by user | Near real time data available (about 1-2 week delay) | General movement trends Connectivity between origin and destination Movement patterns in relation to defined points of interest Gatherings |
Operator Data (cell service providers) | Cell tower density dependent Denser towers produce higher accuracy Varies across provinces and territories (i.e. different urban vs. rural make up) | Sample size dependent on the number of provider subscribers Rare and small fluctuations | Triggered regularly by cell towers for devices receiving a signal and by cell phone events (i.e. calls, texts, emails) | Real time data available (about 2-7 day delay) | General movement trends Connectivity between origin and destination Movement patterns in relation to defined points of interest |
SPOTLIGHT: Privacy & Security of Mobility Data
Contributed by BlueDot Inc.
Ensuring data privacy and security is critically important to BlueDot and requires constant attention in all interactions with clients and data vendors. The privacy considerations that go into data procurement and preparation by BlueDot provide lessons for others working with similar data.
Considerations for selecting a vendor
Safeguarding privacy begins with vetting data vendors. Data providers are not equal in their attention to privacy measures and some are based in countries with less stringent privacy laws than Canada or global leaders in data privacy, like California.2 Although many factors influence the selection of a vendor, privacy considerations that should influence this decision include:
- Adherence to industry-recognized privacy laws, with equal application across all data
- Clear security audits, certifications, and practices
- Clear opt-out and consent abilities for individual device or application users
- Clear limitations and regulations on use of the data being provided
Some important limitations on data use include requirements to dispose of data when a contract ends, minimum thresholds for data aggregation, vendor management of de-identification processes, and restrictions that prevent attempts to reidentify data.
Additional measures to support privacy
Building on those initial considerations, further measures should be taken to enact privacy best practices. When preparing data for clients, BlueDot verifies that the data received from vendors omit any information about a device owner and instead use an anonymous, randomly generated identifier (ID). The data contain no information about a device other than a time and geolocation associated with the anonymized ID. As well, apps providing location data are limited to those with a reasonable purpose for pulling location information, permission to receive these data (e.g. apps for restaurants, shopping, etc.), and requirements that users opt-in through device location settings or through the app itself.
As part of BlueDot’s contract with data vendors and to maintain privacy and statistical representativeness, the outputs created by BlueDot are aggregated by geographic units (e.g., health region) or units of time (i.e., daily, weekly, or monthly reports). As well, data are suppressed when device counts are too low to ensure statistical validity and accuracy. This additional measure further supports privacy and should be done whenever working with these data.
Upholding privacy standards benefits public health
Maintaining high privacy standards is not just a matter of principle; it is crucial for generating meaningful insights that can advance public health. Epidemics are influenced by the behaviours of populations over time, such as whether populations are more or less mobile, the changing connectivity between locations, and the extent to which policy decisions, public health communication and epidemic growth are reflected in behaviour changes. This is why adherence to high standards of data aggregation not only protects the privacy of device users, it is the appropriate level of public health analyses which can contribute to policy insights.
BlueDot Inc.
BlueDot is a Toronto-based infectious disease intelligence company specializing in the application of artificial intelligence (AI) to infectious disease surveillance and risk assessment. Founded in 2013, BlueDot developed an epidemic intelligence platform to support timely responses to infectious diseases.
BlueDot procures and analyzes diverse datasets from publicly and commercially available sources to detect signals of outbreaks around the world at their earliest stages, forecast their patterns of spread through a global network of flights, and support local responses that mitigate their health, economic, and social consequences.
The COVID-19 pandemic led to noteworthy public health applications of BlueDot’s technology, including early detection of the Wuhan outbreak in December 2019 and accurate forecasting of global dispersion of COVD-19, as reported in a peer- reviewed study (2).
2. BlueDot Inc. adheres to Canada’s Personal Information Protection and Electronic Documents Act (PIPEDA) as well as industry-leading policies including the California Consumer Privacy Act and the European Union’s General Data Protection Regulation.
Exploring Data, Developing Goals
To begin work with a novel data source, the PHAC team had to build familiarity with the data and gain expertise for appropriate analyses. They began by asking themselves, What public health questions could these data answer? By exploring likely use cases and learning more about the data’s limitations, their goals for use of the data took shape.
In the context of a global pandemic, PHAC began with hopes for what information could be gained from mobility data and with caution for what ethical concerns might arise. Although the data could not provide information on whether individuals were complying with public health policies—staying two metres apart or self-isolating—it might gauge general uptake in the
population of stay-at-home policies. The de-identified and aggregated mobility data acquired by PHAC would only reflect mass movements of populations and would not provide information on individuals.
The team’s analysts took some time to learn how to work with the very large and growing data sets and how to interpret their representation of peoples’ movements. Early in the project, the team looked at crowdsourced and operator mobility data types and asked, Would both data sets tell a similar story about the movements of Canadians? The dataset analysis compared the same indicator (general movement) over time at national, provincial or territorial, and health region levels. The analysts then posed the question, Were both datasets showing movement patterns of the population increasing and decreasing at the same times? These comparisons showed similar trend lines and helped to validate the data, giving the team more confidence in what they could say about population movements.
Early in the pandemic, the PHAC team had hoped mobility data would help predict where outbreaks were likely to occur and where resources should be deployed to prevent the spread of disease. However, as the rate and complexity of community transmission quickly grew, the data became less useful for prediction and prevention.
As the epidemiology of COVID-19 changed, the needs and priorities for analysis shifted. Through regular touchpoints with COVD surveillance leads, the team gained perspectives that helped guide the analysis. With input from those responding to the pandemic, the team determined that a more appropriate use of mobility data would be to understand historic population movement patterns and trends over the course of the pandemic, and in relation to public health measures enacted in the provinces or at the federal level.
Assessing Mobility Data
Mobility data are very different from public health’s traditional data sources and raise some new opportunities, as well as challenges. After working with mobile device data during the pandemic response, the PHAC team considered the strengths and limitations of these data. Key points from their assessment follow, which may help others to understand some strengths and limitations of similar data from a public health user’s point of view.
Data Strengths & Potential
A valid measure
In order to better understand the validity of their data, PHAC’s team consulted both research and grey literature, which showed mobile device data to be a reasonably accurate measure of population movements (3). The findings of diverse experts engaged within the Agency, other government departments, and non-governmental organizations also contributed to positive assessments of the data’s validity. The immense volume of mobility data is understood to improve validity because the effect of sampling bias is reduced. The data are also less affected by social desirability and recall bias than other sources, such as survey data.
Near real-time information
Mobile device data provide near-real-time information, which the PHAC team saw as helpful for decision-making. Crowdsourced data generally has a 1-week lag time to allow for processing, whereas operator data are available the next day. A near real-time reflection of current circumstances can help public health prepare a timely response to an infectious disease outbreak.
Use in modelling research
Mobility data can be useful for some types of disease modelling that the PHAC team explored. Models that combine information on how the population is moving with other data sets, such as hospital admissions, vaccinations, and disease rates, can help public health validate assumptions or predict where infectious diseases will spread.
Cost efficiency
Because of the large volumes of data obtained, mobile device data can be more cost efficient when compared to survey data that provide relatively small samples, especially for national-level data analyses that PHAC was able to complete in-house. As well, the person-hours required to develop, implement and analyze mobility data were low.
Data Limitations & Challenges
Perceived risk
Public perception that the government might use cell phone data to track people’s movements raises concerns in public health about negative media attention and could discourage use of mobility data. The PHAC team explains to stakeholders that the value of this very large data for public health is not in the individual record, but in the population-level movements, particularly how they change over time. Still, the secondary use of big data is not well understood and combatting misinformation is a challenge. As well, the complex methods used to deidentify and aggregate data for use in public health made it difficult to explain the privacy safeguards for their data to others with limited technical knowledge.
Resourcing the work
Although more cost efficient than other survey methods, it can be expensive to purchase and work with big data, although some free sources are available. The costs are likely to include added staff with specialized skills to work with the data. As well, high and continually growing volumes of big data require a robust computational infrastructure, including additional servers, fast processors, a cloud platform, and a large digital storage capacity. PHAC’s team emphasized that without these resources, their analysis of mobile device data would have been inefficient or impossible.
Need for in-house expertise
Public health organizations may lack staff with training and experience in computing and analyzing big data. Although consultants from the private sector can fill a gap and collaboration with other organizations is essential, nesting technical skills within the team has advantages. PHAC opted to hire staff with the necessary training and experience who contributed to a team that could be dedicated to this work. Having in-house expertise allowed public health perspectives to guide analysis appropriately and provided assurance that public health ethics and valuation of risk versus benefit were applied.
Lack of socio-demographic information
Because mobility data are aggregated and de-identified, it is not possible to link the data to socio-demographic attributes such as age, gender or ethnicity, which limits public health’s ability to understand differences in mobility patterns within the population. The team recognized that this limits the availability of information for developing tailored responses.
Representativeness of sub-populations
Mobile device data are affected by demographic biases related to their usage. People who don’t own devices or use them less frequently are under-represented in these data, whereas those who use more than one device or engage with their device(s) more frequently are over-represented. The data may be less useful for estimating mobility in regions with certain socio-demographic characteristics, such as a high average age. Mobility data also tend to be less representative of remote and rural populations as the sample sizes are smaller for most of these geographies (e.g. Northwest Territories, Yukon, Nunavut). PHAC also noted that the providers of crowdsourced data do not share information on the various apps from which their location data are gathered. This may introduce another source of bias, where data purchasers cannot know the extent to which their sample is reflective of the population as a whole or of groups with particular app preferences.
Validation challenges
The ability to validate mobility data is limited because there is no gold standard with which to compare the data. The PHAC team cautioned that it is not possible to verify that people have moved, but rather the data are a proxy for human movement. This is unlike other secondary data sources used by public health which do allow some verification; with administrative health data, for example, it is possible to check charts to verify hospitalization or which treatments were administered.
Drawing causal inferences
Finally, the PHAC team acknowledged that a key limitation of mobility data is that it is not possible to make causal inferences about population movements based on these data, although correlations may be demonstrated.
Making Use of Mobility Data
The PHAC team explored several use cases for mobility data to provide insight on pandemic policies. The following examples range from a strong use case that proved helpful in their work, to a promising use case that was tested with a subset of data, and a third potential use case, which may be applied in the future.
Strong Use Case
Public health measures and population movement
Mobility data were very helpful for evaluating public health measures through their impact on population movement. During the pandemic, provinces and territories used a variety of public health measures or policies at different times, in response to their local epidemiology. The Stringency Index (see text box) is used to represent the response level employed and can be mapped with COVID-19 disease outcomes data to flag interventions that could be having an effect.
PHAC was able to compare the effects of increasing or decreasing stringency of public health measures on any associated increase or decrease in population movement (see chart below). This provided insights into how the population was responding to changes in public health measures. It was also interesting to see that the associated changes in movement were very clear early in the pandemic, but became less noticeable later in the response. This may have reflected pandemic fatigue or increased confidence in immunity, either due to vaccination or recent recovery from illness.
A limitation of the stringency index is that it can be influenced by the number of large cities in a jurisdiction because they tend to have more severe restrictions than smaller towns or regions (5). Future studies could look at applying the data to more targeted geographic areas to help minimize the impact of large cities. Different elements of the stringency index could also be compared to mobility to identify which individual policies were most effective at limiting population movement.
The Stringency Index
The COVID-19 Stringency Index, developed by the University of Oxford, records the strictness of COVID-19 public health measures and policies that primarily limit people’s behaviour (4).
The index is a semi-quantitative measure that combines information from nine different public health interventions: school closure, workplace closure, cancelation of public events, restrictions on gathering size, public transport closure, stay-at-home requirements, restrictions on internal movement (i.e. within the country or other jurisdiction), restrictions on international travel, and public information campaigns.
The stringency index reflects the response level of the strictest sub-region. It does not measure or imply the appropriateness or effectiveness of a response and does not address compliance or adherence to policies and measures.
Proportion of time spent away from primary location compared to the stringency index of public health measures in select Canadian Provinces (January 2020 – December 2021)
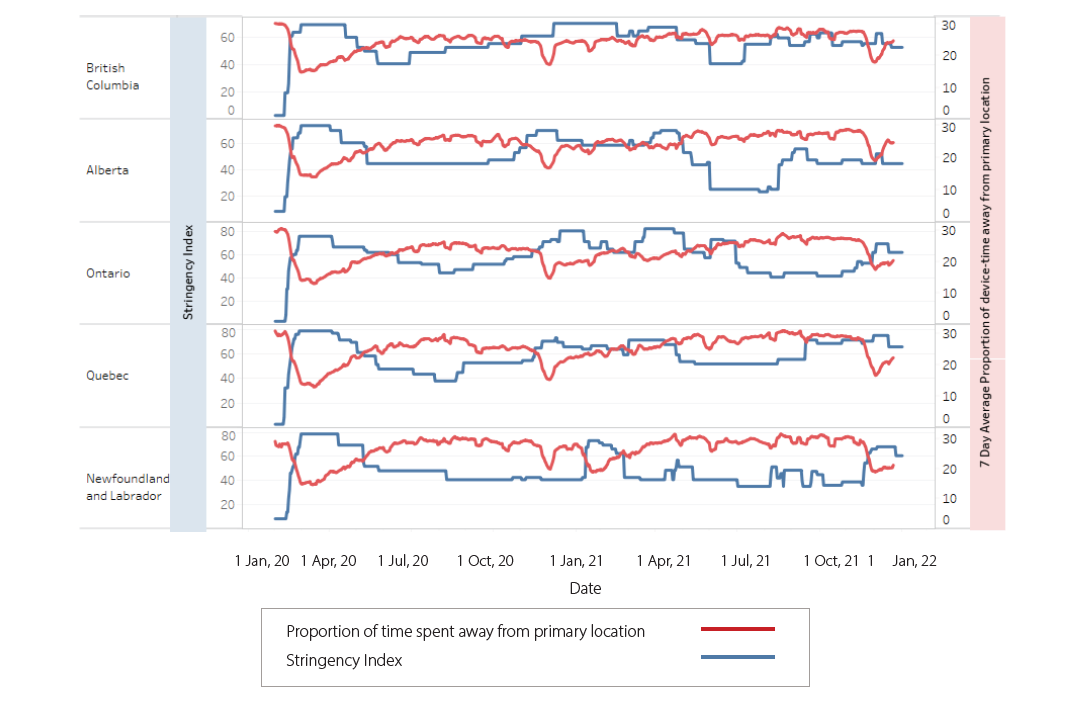
Source note: Public Health Agency of Canada (PHAC) Provincial/Territorial Stringency Index of PUblic Health Measures developed by PHAC-Centre for Food-borne, Environmental and Zoonotic Infectious Diseases (CFEZID), and produced by CFEZID from September 2020 to February 2022, and by PHAC-Centre for Immunization and Respiratory Infectious Diseases (CIRID)-COVID-19 Surveillance Team from February 2022 to present.
Promising Use Case
Movement in high risk regions
Mobility data showed promise for identifying health regions at higher risk of disease transmission within provinces and territories. After loosening public health restrictions, one province experienced an alarming increase in COVID-19 cases. This rapid increase resulted in the implementation of a state of emergency in the province and ‘circuit breakers’ (i.e. no gatherings or travel to other regions) in certain regions to reduce the spread of COVID-19. The measures corresponded with a decrease in mobility across the province and a closer look at zones in the province found that those with circuit breakers also showed the lowest mobility. The example shows that mobility data may be useful for following the outcome of public health action on population movement at a regional level and to understand the impacts of movement at a local level. This application could also be scaled up and built upon to help identify health regions at high risk across the country.
Potential Use Case
Points of interest & behaviour trends
PHAC’s exploration of mobility data suggested that it could offer insights on population behaviour trends, such as visits to particular locations, referred to as ‘points of interest’, including grocery stores, pharmacies, liquor stores, hospitals, long-term care facilities, or organized large gatherings. Mobility trends at points of interest can be analyzed over time periods and compared to previous years. This information can be used to understand trends in relation to public health policies and incidence or prevalence of diseases or risk factors. For example, visits to liquor stores can be an indicator of changes in alcohol consumption in the population, with potential implications for psychological health. Combining mobility trends for all points of interest can also serve as a population-level mobility indicator.
Reflecting on value
These and other use cases explored by PHAC during the COVID-19 pandemic demonstrated value and uptapped potential of big data. The team found that insights from mobility data were particularly useful when the data were combined with information from other sources, including traditional surveillance data. Although mobility data were not directly informing policy, it was a valuable complement that helped fill gaps in knowledge. While the Agency’s exploration of mobility data has focused on COVID-19, more examples of how public health has used these data can be found in the research literature (See the ‘Spotlight’ sidebar).
SPOTLIGHT: Applications of Mobility Data
In the public health research literature
Public health’s use of mobility data has recently focused largely on COVID-19, however, three major areas of use stand out in the literature:
Infectious Disease Public Health
- Mobility data are being used to model population movement, disease spread, hotspots and connectivity between regions, which are illustrated by heat maps and key transmission nodes (6).
- The data have been used in combination with other information, including socioeconomic data—E.g. to understand the relationship between regional income levels and population movement (6,7).
Urban Planning
- Mobility data have most often been used retrospectively, for example, to look at long-term population movements relative to the availability of transportation systems and social services in different locations (8,9).
- As transportation is one of the main sources of carbon emissions, some research has looked at mobility related to transportation modes with a view to how transportation systems could be altered to reduce emissions and combat climate change (10).
Environmental Disaster Preparedness
- Mobility data have been used to determine where resources should be allocated in preparation for emergencies and how
to improve the efficiency of resource distribution (11).
The Take Aways
The Discovery Team considered lessons learned from working with mobility data—what worked well and what might they have done differently. These take-aways can help others understand what to expect and how to plan big data projects.
Gain expert advice, e.g. on legalities and ethics
Because big data are complex and sensitive, public health can benefit from professional advice for a careful review of the ethical, privacy, legal and security implications
of using these data. For example, it can be helpful to understand the difference between legal and ethical responsibilities when purchasing big data.
Inform the public about plans to use big data
It is important to be transparent about plans to use big data and to be precise in explaining where the data come from. A public announcement can use plain language, refer to an intended use case, and explain the expected benefits for public health. It is also helpful to include information about options available to the public, for example, how people can opt out of data collection.
Ensure adequate resources and capacity
A plan to work with big data requires thoughtful consideration of both the technical and human resources needed. The work may require a substantial budget to allow for the purchase of new computers with fast processing capability. Big data projects also require staff with certain technical skills and experience working with big data. Ideally, the team will be multi-disciplinary, including public health program and policy professionals, epidemiologists, data scientists, statisticians and modellers.
Network with and learn from early adopters
Working with new and innovative data can lead to unproductive lines of inquiry or irrelevant analysis. The exploration of non-traditional data is imperfect and requires an openness to experimentation that may fail to meet your needs. To lessen the risk of this unpredictability, public health can invest time in collaborative work with big data. Talking to others with experience analyzing similar data can give you a good idea of the potential of your data—learn what has worked well for them to save your time and effort. Having a multi-disciplinary network of professional colleagues who openly and critically appraise the technical potential and public health value of big data can be particularly valuable in this rather new area of data applications for public health.
Prepare an analytical plan
Although data analysts can do a lot with big data, it is important that an analysis is useful for answering key public health questions and provides information that public health can act on. Developing a data analysis plan—an idea of how the data will be used—can be helpful. The plan can build on what you learn from others about what works.
Support data management processes
The unwieldy size, continual growth and license terms that may apply to big data sets make it all the more important to establish a data governance system. It can be helpful to assign one member of the team to take responsibility for stewarding the data through each step of the data life cycle—from procurement and set up of storage structures, to the implementation of protocols for data access within your organization, to archiving and proper disposal. Preparatory steps and the need for ongoing diligence in data management are often overlooked, but have long-term value.
More to learn
This story represents some of PHAC’s experiences learning about and exploring the potential of mobility data early in the COVID-19 pandemic. Although the Agency has gained new knowledge since this time, the early lessons learned may be helpful for other public health organizations weighing the opportunities and challenges of similar work.
The PHAC team and colleagues now raise questions about what more is possible. Could mobility data be used to describe pre- and post-pandemic activity levels, patterns in population-level movements and transportation modes in different regions, and their relationship to chronic disease rates? What could mobility data tell us about patterns in human movement in relation to the range of infectious disease vectors to predict the spread of viruses related to climate change? What could we learn about food insecurity by looking at mobility in relation to the location of grocery stores within different communities?
The use of mobility and other big data is still quite new in Canadian public health. Although the COVID-19 pandemic sparked innovation and collaboration, including some unanticipated use of big data, there is more to explore.
Acknowledgements
NCCID is hosted by the University of Manitoba. We acknowledge that Treaty 1 territory and the land on which we gather is the traditional territory of Anishinaabeg, Cree, Oji-Cree, Dakota and Dene Peoples, and is the homeland of the Métis Nation.
NCCID extends thanks to members of the Discovery Team for openly sharing their perspectives and lessons learned about the use of big data for public health purposes, as well as for their significant investment of time and valued contributions throughout the development of the case study.
Thanks also go to the Epidemic Intelligence and Data Systems Development teams with BlueDot Inc. for contributing valuable content on data privacy and security, and for their input on other technical content.
The Discovery Team would like to thank the many epidemiologists, researchers, and technical experts from across the Public Health Agency of Canada and Innovation, Science and Economic Development Canada’s Communications Research Centre who gave their time, knowledge and energy to help make sense of these massive data, during a pandemic when everyone was juggling many priorities.
This case study was prepared by Harpa Isfeld-Kiely with the NCCID and developed in collaboration with members of the Discovery Team from the Centre for Data Management, Innovation and Analytics at the Public Health Agency of Canada, with material contributed by BlueDot Inc.