The purpose of this work is to conduct a preliminary analysis of COVID-19 vaccine-related English tweets posted by Canadian users to identify key topics that dominate the conversation and examine the public sentiments regarding these different topics over a short study period. This research was supported by the NCCID 2023 KT Boost Student Awards.
Authors
Hassan Maleki Golandouz, Wendy Xie, Lisa M. Lix
Introduction
The vaccination rollout was vital for protecting Canadians against severe outcomes from COVID-19 infection, and social media platforms like Twitter have been recognized as potentially valuable sources of information about the public’s perceptions of vaccines. This is a preliminary study to analyze COVID-19 vaccine-related English tweets posted by Canadian users over a short study period to identify key topics that dominate the conversation and to examine the public sentiments related to the identified topics.
Methods
This study focused on vaccine-related English tweets posted by Canadian users over a span of five weeks between November and December 2021. During this pivotal period, the Pfizer-BioNTech COVID-19 vaccine for children aged 5 to 11 was approved by Health Canada and a federal vaccination mandate for employees was implemented. The data was sourced from the public domain and was compiled using Netlytic, a cloud-based tool adept at automating the collection of tweets via Twitter’s public API, with a geographical focus on Canadian locations as specified in Twitter user profiles.
To identify key vaccine-related topics during the study period, the text was pre-processed for topic modeling through a series of steps: removal of punctuation, tokenization, elimination of stop words, and lemmatization, as depicted in Figure 1. We then applied Correlation Explanation (CorEx) topic modeling, a technique proficient in unraveling primary topics by maximizing mutual information, or total correlation, between words and underlying topics. This method intricately explores correlations within tweets, highlighting specific words, phrases, and broader themes that are instrumental in identifying key latent topics. The process continued until the emergence of additional topics no longer offered a significant increase in total correlation.
To conduct sentiment analysis, we utilized the Valence Aware Dictionary and Sentiment Reasoner (VADER) to deduce sentiment scores. VADER is finely tuned to the nuances of social media sentiments and categorizes sentiments into three distinct classifications: Positive (with a score of ≥ 0.05), Neutral (where -0.05 < score < 0.05), and Negative (when the score is ≤ -0.05).
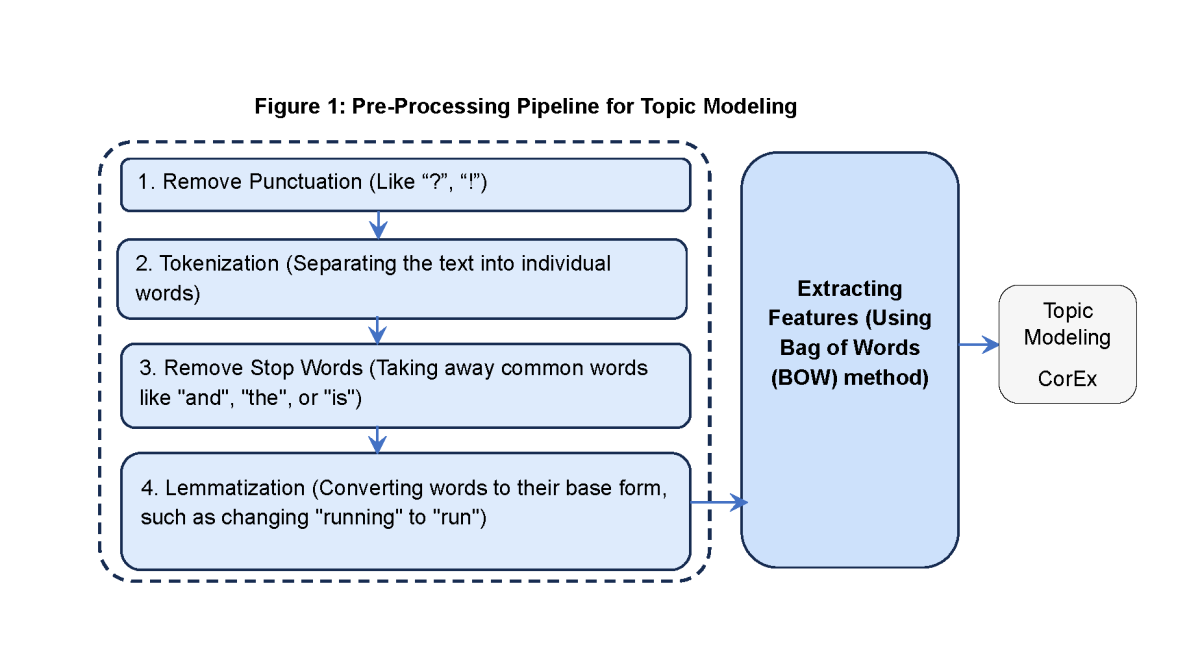
Results
After the exclusion of retweets, the dataset was narrowed down to 8,521 COVID-19 vaccine-related tweets originating from 4,587 users. A total of 17 distinct topics were discerned through the application of CorEx topic modeling, some of which included vaccine appointments, vaccination social movements, vaccine legalities, and more (Figure 2). An analysis of sentiment scores using VADER revealed a similarity between positive and negative sentiments across the majority of topics (13 of 17 topics). Topics that exhibited the most significant disparities in sentiment were related to vaccine and healthcare staff (43% negative), child vaccination (46% positive), vaccine approval (50% positive), and vaccine appointments (38% positive) (Table 1).
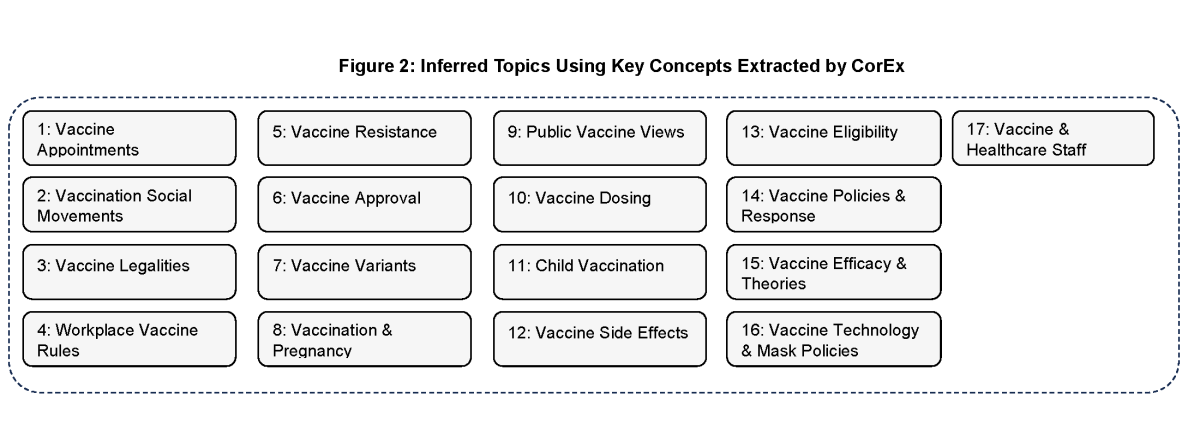
Table 1. Valence Aware Dictionary and Sentiment Reasoner (VADER) was used to categorize sentiment scores into three distinct classifications: Positive, Neutral, and Negative. Four of the topics resulted in overall negative (vaccine and healthcare staff) or overall positive (child vaccination, vaccine approval, vaccine appointments) sentiments.
Topic | Positive | Negative | Neutral |
Vaccine and healthcare staff | 30% | 43% | 26% |
Child vaccination | 46% | 23% | 30% |
Vaccine approval | 50% | 37% | 13% |
Vaccine appointments | 38% | 26% | 36% |
Discussion
This work demonstrates how public health policymakers can utilize sentiment analysis methods as strategic tools to analyze tweets during and following significant policy shifts. This work highlights how a sentiment analysis approach can serve two primary purposes: first, it can allow policymakers to leverage information from a spectrum of viewpoints across Canada, enabling a gauge of public sentiment. Second, it can provide a robust foundation upon which to strategically develop and adapt vaccination and educational programs, ensuring they are responsive to the public’s evolving concerns and needs.